035 - Bob Pardo Part II - Building Trading Strategies that Work with Walk Forward Analysis - Part 2 of 2
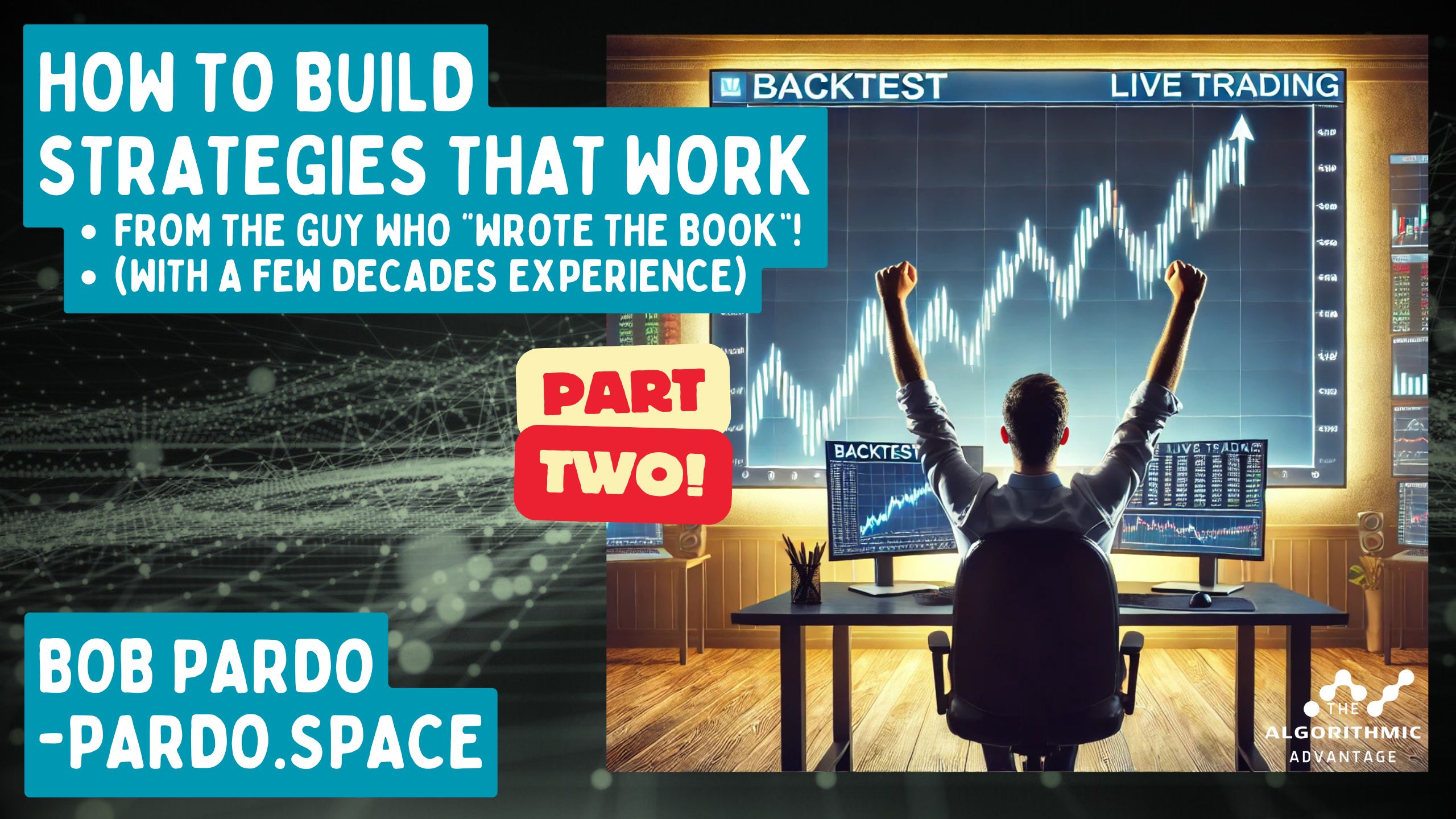
Building Back Tests that match Live Performance
I had a thought this week about what constitutes my "trading edge". You know, the question every trader is expected to be able to answer. It's supposed to constitute some kind of evidence that you can out-perform the market, your peers, or whatever. Something Bob Pardo mentioned made me think differently about this when he reminded me that when trading pits were around, every trader "had to have their edge" to stay ahead of the other guy or gal. Back then, on the floor, it was necessary to have some kind of "insider knowledge" so to speak in order to carve out success. But perhaps times have changed, and this phrase doesn't even carry the same weight any longer? I mean, when I think about what I have that enables me to generate great returns, it's a combination of a lot of experience, hard work, generating good processes, training the brain to think a certain way, building skills through constant education, using the right tools, finding the right technology, making sure I have first class execution & data, and so on. It's that I treat trading as a business, one with very fine margins, and I strategize like any good businessperson to find my place in the market.
So is it any different to being a good engineer? Surgeon? Farmer? Isn't it sufficient to just commit to something long enough, keep getting up after knockdowns, train harder, study more, practice, and eventually, become "good enough" to be a master at your trade? I'm not trying to win a gold medal; I'm trying to generate sufficient success to make a living. "How can I out-perform my peers then" one might ask. I'm not trying to! I'm happy to match them. I just want to be in that percentile of traders who do really well, over the long term. In the process, I may out-perform a lot of the plain vanilla funds, but there's a reason for that: they have specific motivations, are driven by different (peer-and-market-following) mandates, restricted by trading enormous amounts of capital, use outdated tools, have to wade through piles of corporate red tape and regulatory burdens, etc., etc.
I mention this because when you talk to a guy who has spent decades on the craft, and carved out amazing results along the way, the over-riding theme is the refinement and enhancement of the process over time. Developing the right process, using the right tools, applying the right mindset, gosh, these are an edge right. These are the things to be working on. You don't have to be born with it, although it helps if you've got the right personality, for sure. That's another topic we'll get into with Brett Steenbarger when we talk to him on the show soon. So, I think the good news is that with a podcast like this, you get a free masterclass from the masters, and you should invest the time in making the most of it. If there's better questions I could ask, or people you'd like me to talk to, heck, just email me, I'll get on it.
What Is Walk-Forward Analysis?
Walk-forward analysis (WFA) is a systematic method to validate trading strategies by iteratively optimizing parameters on in-sample data and testing performance on subsequent out-of-sample data. Unlike traditional optimization, WFA simulates real-world trading by "rolling" the optimization window forward over time. This generates an entire back-test out of out-of-sample results, seeking to be scientific and systematic about developing strategies which will adapt to evolving market conditions while avoiding overfitting.
Key Principles for Robust Strategy Development
You must still validate: There are still traps, and art and science must still meet. A single walk-forward test may not be enough. Explore ways to use the WFA method. Apply the scientific method to use the tool to explore the data. It's going to take targeted effort to work out how to make WFA work for the time-frame and strategy-type you are developing.
Assessing the results: Profitability alone isn’t sufficient. Look for stable performance across all out-of-sample windows, be aware of regime shifts, trade counts, specific strategy strengths and weaknesses. Explore different time periods, different objective functions, and so on.
Avoid curve-fitting: WFA exposes over-optimized strategies. If parameters spike in isolated windows but fail elsewhere, the strategy is likely fragile.
Objective function design: Prioritize risk-adjusted metrics (e.g., profit/drawdown) over raw returns. Bob emphasizes tools that assess equity curve smoothness and trade distribution. Reject parameters that work only in narrow ranges. Seek "plateaus" where neighboring values perform similarly.
Regime awareness: Understand that strategies are tied to the data they’re built on. Test across bull, bear, and sideways markets to ensure adaptability.
Sample size sensitivity: High-frequency strategies naturally offer more data, but longer-term approaches (e.g., trend-following) require careful window sizing or bespoke methods to generate the needed sample size. Consider testing on other markets.
Risk management: The iterative process highlights variations in trade frequency, drawdowns, and profit consistency, allowing traders to refine their strategies and manage risk more effectively.
Final Takeaways
Bob’s framework prioritizes adaptability and empirical rigor. By combining WFA with curated tools (e.g., Ranger) and disciplined evaluation, traders can:
- Identify strategies that perform consistently across regimes.
- Minimize overfitting risks inherent in traditional optimization.
- Build diversified portfolios of uncorrelated strategies for smoother equity curves.
- Have a built-in process for on-going monitoring and updates of strategies.
For advanced traders, the key lies in balancing automation with strategic intuition—leveraging WFA to validate ideas while respecting market complexity.
Get in Touch with Bob